It wasn't long ago that consumers distrusted online commerce. Would credit card numbers be stolen? IDs hacked? Few wanted to find out. Today, e-commerce is ubiquitous, with customers trusting online retailers with sensitive personal and financial data. A similar trajectory faces artificial intelligence as it advances into sensitive domains like healthcare, law enforcement, finance, and transportation.
Trust in the Age of AI
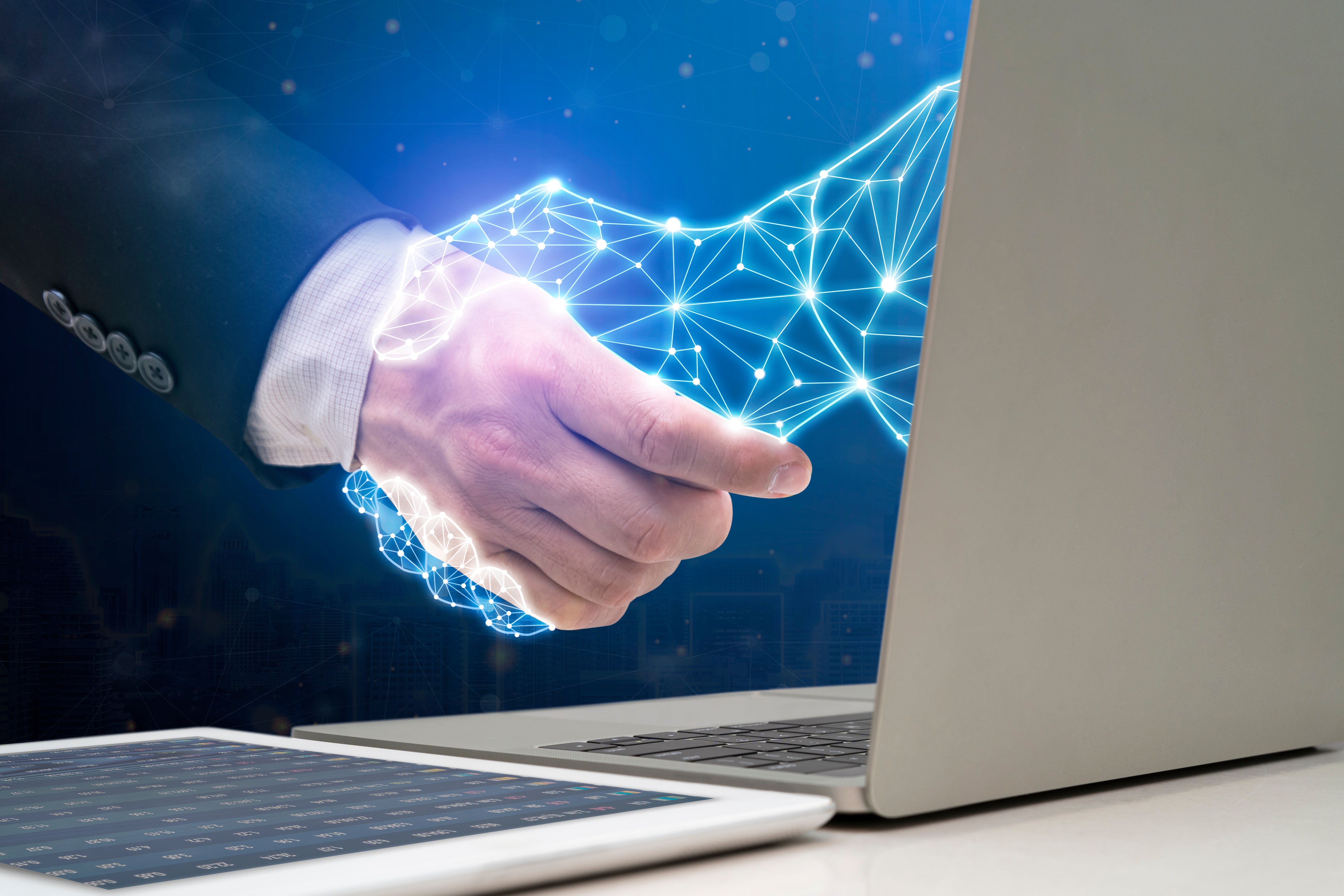
Recent surveys reveal around half of consumers feel wary about AI driving cars, handling finances, diagnosing illness, or surveilling public spaces (1). High-profile mishaps have amplified skepticism. In 2016, Microsoft’s AI chatbot Tay turned racist on Twitter, spouting hate speech after trolls provoked it (2). In 2017, an algorithm used by UK courts and police to predict re-offending was found biased against people of color, falsely labeling them as higher risk (3). In 2018, an Uber autonomous car struck and killed a woman in Arizona, startlingly demonstrating the safety risks of self-driving vehicles (4).
These incidents illuminate challenging issues around AI accountability, security, ethics, and transparency. However, industry, government, and academia are undertaking concerted efforts to build public trust by addressing such concerns. Groups like the Partnership on AI and the Institute for Ethical AI promote policies and best practices for the responsible design and deployment of AI systems, backed by major tech firms and AI experts (5). Governments have established advisory councils on AI adoption and proposed regulations mandating risk assessments, transparency, and non-discrimination in algorithmic systems (6).
Technical initiatives also aim to “open the black box” of AI decision-making. Explainable AI techniques shed light on why algorithms make specific predictions or recommendations, enabling audits for accuracy and fairness. For example, the software can generate natural language explanations of the key data features that led an AI loan approval system to deny an application (7). Formal verification uses mathematical logic to prove an AI behaves as intended. For instance, by modeling traffic laws, verification can prove autonomous vehicles satisfy safety requirements in a wide range of simulated driving scenarios (8).
Adversarial techniques involve stress testing AI systems against misleading data, hacking attempts, and other attacks to identify vulnerabilities pre-deployment (9). Researchers fooled a self-driving car into misclassifying a stop sign by subtly altering it with stickers, illuminating security flaws (10). Hybrid decision-making incorporates human oversight for approving major AI actions in sensitive domains like medicine and transport, ensuring accountability (11).
Extensive trial evaluation in simulated environments characterizes the strengths and limitations of AI systems before real-world deployment. For autonomous vehicles, billions of test miles are driven in virtual simulators and closed-track facilities to validate safety across diverse conditions (12). AI diagnostic tools are evaluated against thousands of real patient cases to compare accuracy versus experienced physicians (13). Limited trial deployments with human supervisors ready to take over also help characterize performance.
Blockchain technology offers another route to monitor AI systems by tracking data sources, processes, and decisions on an immutable ledger. This audit trail enables explanation when disputes arise over algorithmic decisions or recommendations (14). Cryptographic techniques like homomorphic encryption analyze data while encrypted, enabling AI to make predictions without exposing actual personal contents (15).
Through collaborative efforts on technological, policy, and ethical fronts, a layered approach is emerging to build public trust in artificial intelligence (16). But transparent communication remains critical, avoiding hype and conveying realistic capabilities and limitations.
Much like the early days of online commerce, removing uncertainty around security, accountability and integrity of AI systems can enable societies to confidently adopt them. Other precedents like automobiles, airplanes, and microwaves show that initially novel technologies facing public skepticism can become widely embraced and trusted through advancing innovation paired with appropriate regulation, industry practices, and public education.
For example, cars were once unpredictable novelties on roads shared with horses, garnering fears over safety. But improved manufacturing standards, traffic regulations, licensing requirements, and safety features like seatbelts helped build societal trust in automobiles. Early airplane travel was viewed as risky due to technical failures and accidents. But aviation rapidly advanced through extensive testing, safety protocols like air traffic control, and engineering redundancies.
Consumer uncertainty around microwave ovens and radiation risks was alleviated through studies confirming safety and the establishment of product standards. In each case, persistent advancement in the core technology accompanied by efforts to ensure ethical application, accountability, and public understanding helped transition these innovations from doubt to mass adoption.
Artificial intelligence has made remarkable strides but still faces an era of skepticism. With diligent work on safety, transparency, accountability, and communication of realistic capabilities, AI systems can follow the path of earlier technologies that transformed from uncertainty to general confidence and trust. Just as previous generations came to adopt pioneering innovations like electric lights, telephones, and computers, today's public uncertainty around intelligent machines can similarly give way to acceptance of AI's responsible and beneficial use.
Who knows? Someday, we may wonder how we ever lived without the convenience of having an AI assistant to rely on.
Sources:
- https://www.pewresearch.org/internet/2022/03/17/how-americans-think-about-artificial-intelligence
- https://www.theverge.com/2016/3/24/11297050/tay-microsoft-chatbot-racist
- https://www.theguardian.com/technology/2017/dec/04/racist-facial-recognition-white-coders-black-people-police
- https://www.nytimes.com/2018/03/19/technology/uber-driverless-fatality.html
- https://ethical.institute/principles.html
- https://ec.europa.eu/digital-single-market/en/news/ethics-guidelines-trustworthy-ai
- https://arxiv.org/abs/1710.00794
- https://www.ncbi.nlm.nih.gov/pmc/articles/PMC10181674/
- https://www.linkedin.com/pulse/adversarial-attack-resistance-safeguarding-ai-systems-udayaprakash/
- https://arstechnica.com/cars/2017/09/hacking-street-signs-with-stickers-could-confuse-self-driving-cars/
- https://www.cigionline.org/articles/artificial-intelligence-and-keeping-humans-loop/
- https://www.rand.org/pubs/research_reports/RR1478.html
- https://www.nature.com/articles/s41746-020-00323-1
- https://info.kpmg.us/news-perspectives/technology-innovation/four-ways-blockchain-and-ai-together-can-build-trust.html
- https://iapp.org/news/a/the-latest-in-homomorphic-encryption-a-game-changer-shaping-up/
- https://www.ericsson.com/en/blog/2019/10/8-principles-of-ethics-and-ai